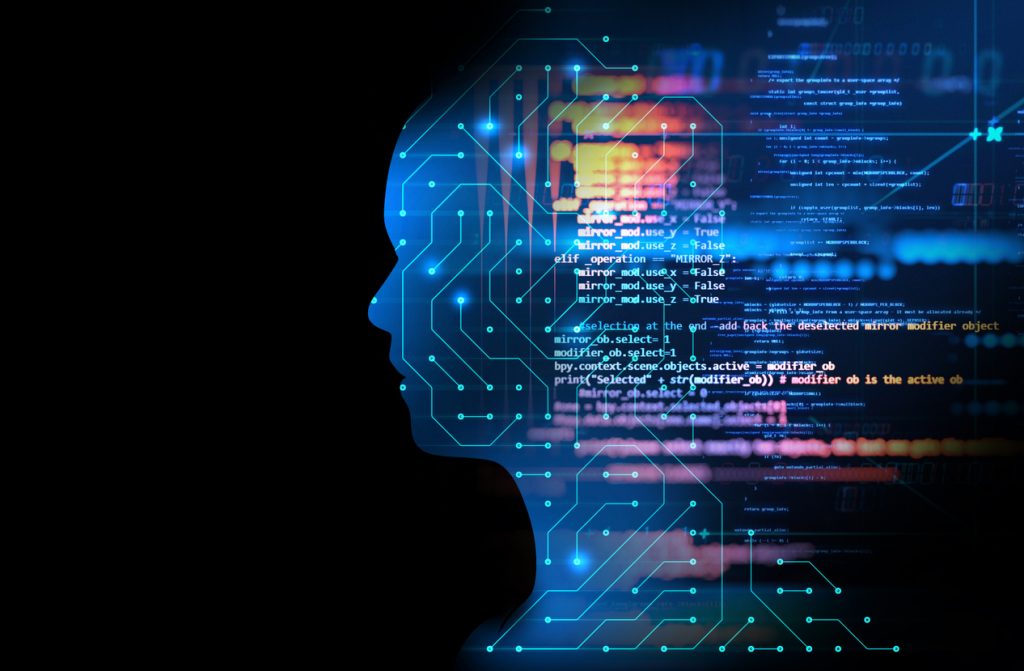
Machine Learning applied to financial services industry has the potential to improve outcomes for both businesses and consumers. And in the UK, firms are beginning to take advantage of this. A recent survey, called ‘Machine Learning in UK Financial Services‘, carried out by the Bank of England (BoE) and the Financial Conduct Authority (FCA) has found that two thirds of respondents report they already use it in some form. The median firm uses live ML applications in two business areas and this is expected to more than double within the next three years.
The Bank of England (BoE) and Financial Conduct Authority (FCA) have a keen interest in the way that ML is being deployed by financial institutions. That is they we conducted a joint survey in 2019 to better understand the current use of ML in UK financial services. The survey was sent to almost 300 firms, including banks, credit brokers, e-money institutions, financial market infrastructure firms, investment managers, insurers, non-bank lenders and principal trading firms, with a total of 106 responses received.
The survey asked about the nature of deployment of ML, the business areas where it is used and the maturity of applications. It also collected information on the technical characteristics of specific ML use cases. Those included how the models were tested and validated, the safeguards built into the software, the types of data and methods used, as well as considerations around benefits, risks, complexity and governance.
Although the survey findings cannot be considered to be statistically representative of the entire UK financial system, they do provide interesting insights.
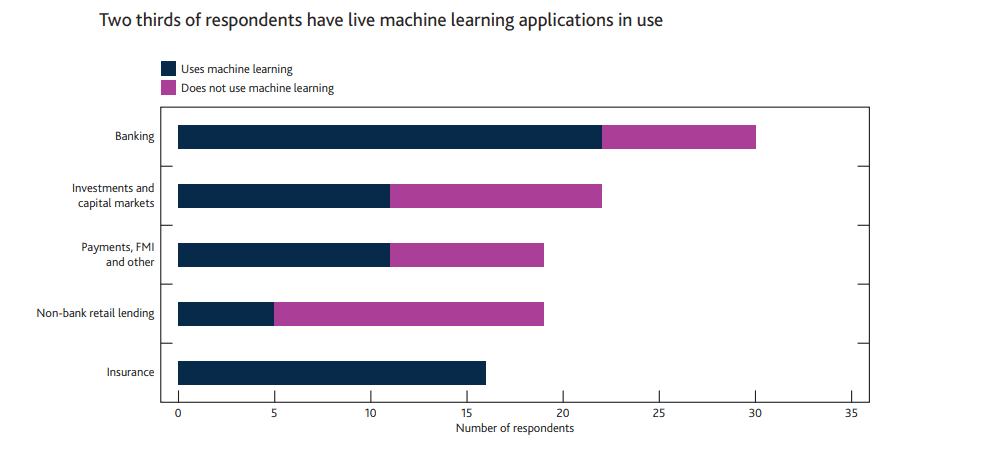
The survey found that in many cases, ML development has passed the initial development phase, and is entering more advanced stages of deployment. It has been proved that one third of ML applications are used for a considerable share of activities in a specific business area. And among all these activities, deployment is most advanced in the banking and insurance sectors. From front-office to back-office, ML is now used across a range of business areas.
Likewise, one of the most commonly uses that financial firms are applying ML to is in anti-money laundering (AML) and fraud detection as well as in customer-facing applications (eg customer services and marketing). Some firms also use ML in areas such as credit risk management, trade pricing and execution, as well as general insurance pricing and underwriting.
Another key fact that the survey found is that regulation is not seen as a barrier but some firms stress the need for additional guidance on how to interpret current regulation. Firms do not think regulation is a barrier to ML deployment. The biggest reported constraints are internal to firms, such as legacy IT systems and data limitations. However, firms stressed that additional guidance around how to interpret current regulation could serve as an enabler for ML deployment.
Regarding potential risks financial services companies may face when applying ML, the survey found that firms thought that ML does not necessarily create new risks, but could be an amplifier of existing ones. Such risks, for instance ML applications not working as intended, may occur if model validation and governance frameworks do not keep pace with technological developments. Firms use a variety of safeguards to manage the risks associated with ML. The most common safeguards are alert systems and so-called ‘human-in-the-loop’ mechanisms. These can be useful for flagging if the model does not work as intended (eg in the case of model drift, which can occur as ML applications are continuously updated and make decisions that are outside their original parameters).
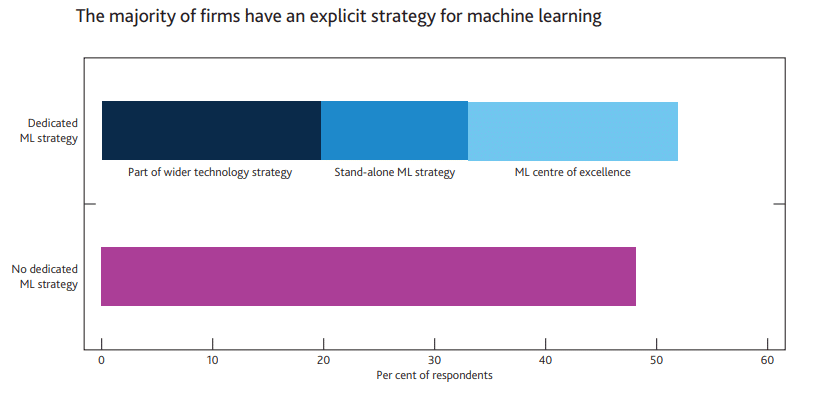
In parallel, firms also validate ML applications before and after deployment. The most common validation methods are outcome-focused monitoring and testing against benchmarks. However, many firms note that ML validation frameworks still need to evolve in line with the nature, scale and complexity of ML applications. Firms mostly design and develop ML applications in-house. However, they sometimes rely on third-party providers for the underlying platforms and infrastructure, such as cloud computing.
The majority of users apply their existing model risk management framework to ML applications. But many highlight that these frameworks might have to evolve in line with increasing maturity and sophistication of ML techniques. This was also highlighted in the BoE’s response to the Future of Finance report. In order to foster further conversation around ML innovation, the BoE and the FCA have announced plans to establish a public-private group to explore some of the questions and technical areas covered in this report.
The promise of ML is to make financial services and markets more efficient, accessible and tailored to consumer needs. At the same time, existing risks may be amplified if governance and controls do not keep pace with technological developments. More broadly, ML also raises profound questions around the use of data, complexity of techniques and the automation of processes, systems and decision-making. And in recent years, improved software and hardware as well as increasing volumes of data have accelerated the pace of ML development, specially in the financial services industry.
Read More:
hedge fund vs asset management
aqr capital management hedge fund
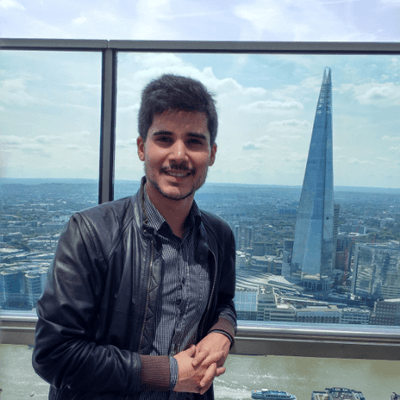
Hernaldo Turrillo is a writer and author specialised in innovation, AI, DLT, SMEs, trading, investing and new trends in technology and business. He has been working for ztudium group since 2017. He is the editor of openbusinesscouncil.org, tradersdna.com, hedgethink.com, and writes regularly for intelligenthq.com, socialmediacouncil.eu. Hernaldo was born in Spain and finally settled in London, United Kingdom, after a few years of personal growth. Hernaldo finished his Journalism bachelor degree in the University of Seville, Spain, and began working as reporter in the newspaper, Europa Sur, writing about Politics and Society. He also worked as community manager and marketing advisor in Los Barrios, Spain. Innovation, technology, politics and economy are his main interests, with special focus on new trends and ethical projects. He enjoys finding himself getting lost in words, explaining what he understands from the world and helping others. Besides a journalist he is also a thinker and proactive in digital transformation strategies. Knowledge and ideas have no limits.